This website uses cookies. See how.
Introduction
Email marketing is unique and cannot be treated like other channels. Unlike display ads, iIt only begins with a sent email that can be opened and read at any time.
This means that you cannot simply sum and report numbers on a given day. Why not? Let's illustrate this with an example:
Imagine running a display advertising campaign with following statistics.
Display adImpressionsClicksCTRDay 11001010%Day 2200105%In general the click through rate for the display ad that will be reported on Day 2 would be 5%.
Email newsletterSendOpenOpen rateDay 11000?Day 2050?A second example
So, what is the open rate on Day 2? 50 unique opens and 0 sends? Of course not. We are unable to report a unique open rate on Day 2 as we cannot relate any sends to the 50 unique opens. We need to relate the 50 unique opens to the 100 sends on Day 1. Fortunately, all email service platforms are generally able to tackle this challenge.
But how do you calculate the send-to-cart ratio or the send-to-checkout ratio? Cart or checkout data typically resides in a web analytics system and is out of the scope of an email service platform (ESP). How do you relate cart or checkout data generated by users who received the newsletter on Day 1 back to the initial send date?
If you want to accurately report CRM, webanalytics (when it's not tracked by the ESP) and email marketing data in relation to each other you need a system or engineer. Treating email marketing data the same as all your other channels will result in inaccurate reporting and this is why each company that takes email marketing seriously should have a single source of truth for email analytics.
So where do you start?
Step 1: Identify the Key Metrics
You are probably thinking, "No sh*t, Sherlock." And we understand you.
Even in the world of data-driven everything, settings clear goals and key performance indicators (KPIs) is not given the emphasis it deserves.
So, to start, we recommend this three-step approach:
- Determine the main secondary objectives for your email marketing activities. Do you want to drive sales, increase leads, increase subscribers, or something else?
- Identify the underlying KPIs/metrics that influence the main or secondary objective. It can help to visualize the relationship of the main objective to the underlying KPIs/metrics in a pyramid-like structure as shown below:
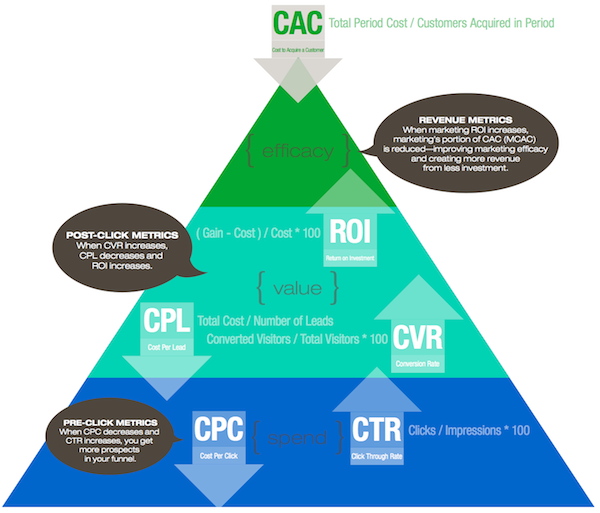
source: https://martech.org/the-digital-marketing-metrics-pyramid/- Prioritize the metrics based on their relevance to the overarching objective and the level of impact they have on the success of your email marketing efforts. This helps you identify the metrics you should monitor more frequently and the metrics which can have a lower priority.
To learn more about metrics, check out our post on 13 key email marketing KPIs.
Step 2: Determine the Data Sources
So you have identified the main and, optional, secondary objectives with the related metrics? The next step is to identify the key data sources.
Below are the top 4 relevant sources you should use for email marketing analytics reporting:
- Email service provider (ESP) data: This includes data on email opens, clicks, bounces, and unsubscribes. And of course this relates to all the dimensions like campaign name, send date, type of mail you want to break down your metrics on. Most ESPs provide this data in their reporting dashboard but the preference here is to have this available through the API.
- Web analytics data: This includes data on website visits, pageviews, and conversions that result from email campaigns. You can track this data using web analytics tools such as Google Analytics, some ESP’s even support tracking of these metrics.
- Customer relationship management (CRM) data: This includes data on customer behavior, preferences, and interactions.
- Sales data: This includes data on revenue generated from email campaigns. You can track this data using your sales system or CRM.
By combining data from these sources, you can get a complete picture of the impact of your email marketing activities on customer behavior.
Important requirements for each data source
Email service provider (ESP) data
Check if your ESP is able to export data on email sends, opens, clicks bounces etc. on preferably an individual send and/or subscriber level through an API. This enables you to automatically connect the data with other sources on a very granular level which in turn enables complete reporting of your email marketing activities.
Marketing automation companies like Klaviyo, Braze, Bloomreach, Deployteq, Copernica and many more email service platforms already provide a data model that enables this.
You can find also find an example on what an individual record from ESP preferably looks like below:
Dimension nameExample valueuuidd58177e0-a209-45cf-b059-ac7939365a16metricclicksmetric_timestamp2022-12-19 21:10:34.000000 UTCmetric_id1226customer_id201email_domaintest.combroadcast_id4962broadcast_typeMAILbroadcast_event_typeQUICKMAILcampaign_id12campaign_nameQuickmail #12campaign_object_id1content_id531subjectThis is a test messagemailing_typetest_mailtest_mailingfalsebroadcast_timestamp2022-02-28 15:06:43.000000 UTCosWindowsbrowserChromelink_id24964url{{viewurl}}google_sourceemailgoogle_mediumtest_mailgoogle_termgoogle_contentgoogle_campaigntest_mail_blogpostmarketUKaudiencenew_usersexternal_id201customer_email_address7d673339b751457e862da284e1d19cdacemail_address7d673339b751457e862da284e1d19cdacemail_address_overridetrueua_deviceDesktopua_osinfo_nameWindowsmachine_generatedfalse
We know it's quite extensive but there is a ton of insightful information hidden in each attribute.
Do not forget to align your exports with your privacy officer if you want to include information related to the individual subscriber! They can advise on what is permitted and what is not.
Web analytics data
For web analytics data, it is important that your email marketing tagging is in order. We will follow up with a separate blog post on tagging best practices.
The blog below already gives you a great start:
https://web.utm.io/blog/utm-parameters-best-practices
Also ensure that all the conversion goals that you would like to include are set up in your webanalytics tracking.
In addition, ensure that your web analytics system has an API to pull data from. Custom tagging parameters, such as the ID of the individual broadcast or send, can also make for a more robust integration with ESP data.
CRM data
CRM data can further enrich your email marketing analytics dataset with attributes that give insights into what kind of customers have received your data. You can include customer segments like segment information from a RFM model or add demographical data like age and gender.
When adding these attributes be mindful to include the attributes in your email tagging. Without them you won't be able to slice and dice your web analytics metrics on these attributes. A general rule of thumb is to add all the crm attributes to your tagging. Integrating your ESP with your CRM this way is the first step to mastering your data.
Sales data
You might wonder why you should include Sales data if you’ve already integrated webanalytics data. Doesn't your webanalytics system also include your sales data?
Including sales data has a lot of benefits:
- Sales data can fill in the gap created by adblockers
- In a potential cookie-less world, sales data can be the one source of truth to attribute sales to e-mail marketing activities if all cookie-based solutions fail. If you're curious how that would work please reach out. This topic deserves a post on its own, one which we will definitely tackle in a future post.
Your sales data at minimum should include a subscriber ID that also lives in your ESP to be able to integrate the data with your ESP data.
Step 4: Integrate Data Sources with the Platform
Once you have identified your data sources, the next step is to combine them into one dataset to enable analysis.
Of course, we recommend doing this in SEINō.
There are, other solutions that you could consider, which we describe further down in this paragraph. But before getting into that, please first inventory the following:
How can I get data out of the source system? Common ways of exporting data from source systems are:
- Manual CSV/Excel exports
- API exports (recommended)
- Export to FTP location
- Automated email exports with attachments
Also, check the limitations of each export type. Most vendors limit the export to a certain number of records or a maximum file size that you can export at a given time.
A web analytics system like Google Analytics is notorious for sampling data via the API when processing high volumes of data. This, in turn, can affect your data quality.
Google Analytics has a great documentation if you are unfamiliar with sampling and how it affects your data reporting:
https://support.google.com/analytics/answer/2637192?hl=en#zippy=%2Cin-this-article
Next, what can you export out of the system? Are all fields that you require to build your email analytics dataset also available for exporting? We’ve experienced first-hand that the fields that are available within your source system are not necessarily available when exporting data.
What format can I export? Is the platform where I will integrate my data able to process this data format? When we talk about data format, we are referring to file formats such as CSV, JSON, or Excel files.
So what solutions are there to combine the data sources in one email analytics dataset? In our view, besides SEINō, you can explore the following routes:
- The cumbersome route of working with Excel and using lookups to join tables of data. This is definitely not scalable and we don’t recommend it. Seriously, don’t do this.
- Integrate your data sources in a data warehouse system like BigQuery or Snowflake where you combine your data sources via scripting.
- Integrate your data sources in a customer data platform like Bloomreach or Tealium.
Let’s quickly sum up the pros and cons for the last two options. We really think the first option is not worth considering.
Integrate your data sources in a data warehouse system like BigQuery
Pros:
- Scalability: A data warehouse system is meant to process large volumes of data with ease. So if you’re mailing frequently and processing huge volumes of data, you're future-proofing yourself with this option.
- Speed: In gathering insights, speed is of the utmost importance. You do not want to wait hours or even minutes before your query returns a result.
- Flexibility: Most systems similar to BigQuery support a wide range of data formats and numerous ways of onboarding external data within the Cloud provided ecosystem.
- Cost-effective: Most cloud-hosted warehouse systems operate on a pay-for-usage model.
Cons:
- Complexity: A data warehouse system generally requires specialized skills to operate. Users need to be familiar with SQL and distributed computing concepts. In our experience, most CRM or email marketing teams do not have direct access to these resources.
- Data onboarding: Getting the data into a data warehouse in an automated fashion also requires specialized skills. Users have to set up processes via scripting to onboard, validate, clean, and store data.
- Management overhead: Although cloud data warehouse systems cover and support you in many things, you will still have to monitor your costs and the performance of your data pipelines to ensure efficient usage.
- Reporting: Most data warehouse systems will require an additional solution to report and/or analyze the data. Tools like Looker or Tableau could be used but also require specialized skills and extra time and effort to set up.
Customer Data Platform (CDP)
Pros:
- Pre-built integrations: Customer Data Platforms offer libraries of pre-built integrations which, in most cases, are encased in user-friendly widgets wherein the end-user can set up an integration within a few clicks.
- Data unification: A Customer Data Platform's primary function is the consolidation and unification of (customer) data. This is a major benefit as all the hard work of concocting the required queries has already been done for you.
- Managed solution: A Customer Data Platform manages your entire data pipeline. So you will have less overhead in managing your solution.
Cons:
- Cost: The implementation and recurring cost of a Customer Data Platform can be quite steep, and not every company is able to make a positive business case.
- Reporting capabilities: Not all CDPs are focused on providing strong reporting or analytical capabilities. There are Customer Data Platforms that do, like Bloomreach. Most Customer Data Platforms focus on unification and can expose unified datasets to specialized reporting tools.
In the next post we will continue the topic and write about the importance of cleansing and validating your data and email analytics analysis.
See SEINō in action and take your email reporting to the next level